Navigating the AI Landscape and Power Struggles
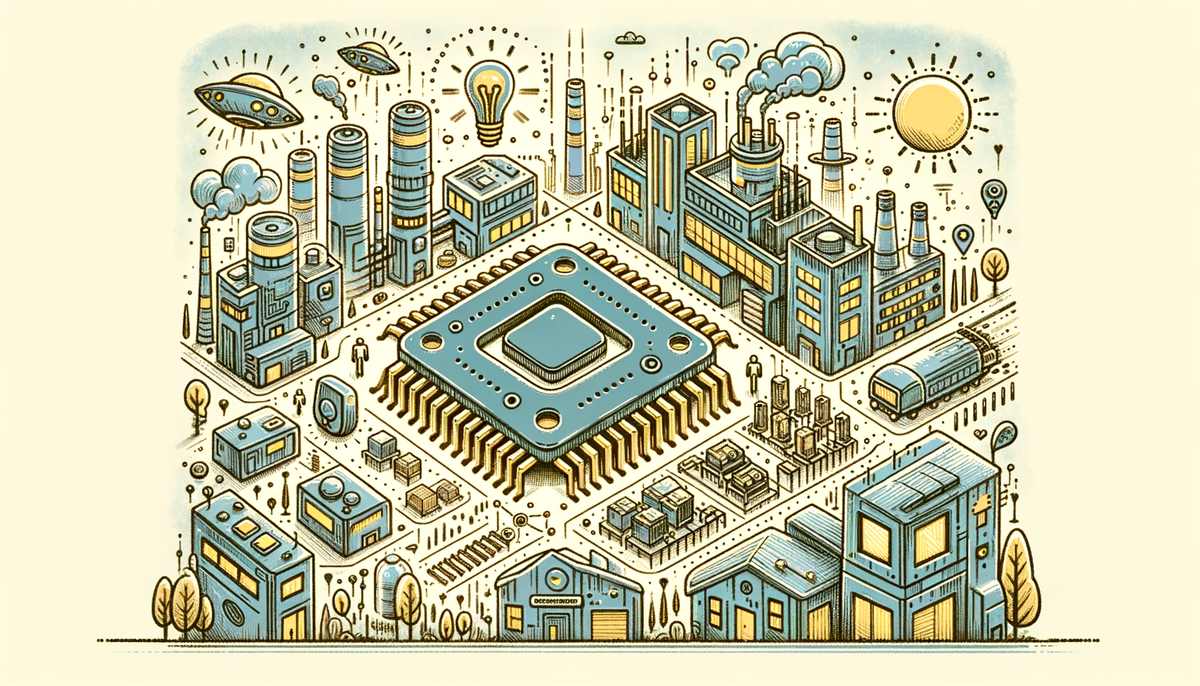
When a custom-built chip sparks a potential shift in industry dominance, it challenges not only market leaders but the very future of technology itself.
Meta’s Bold Move: Engineering a New Era in AI Hardware
Meta is betting big on reshaping its AI infrastructure with a custom-made training chip aimed squarely at disrupting Nvidia’s grip on the market. With projected AI capital expenditures reaching around $65 billion in 2025, Meta’s ambition is palpable. This isn’t just about lowering costs; it’s about carving out a clearer path to efficiency and independence from Nvidia’s expensive GPUs. By teaming up with Taiwan Semiconductor Manufacturing Company (TSMC), Meta has set the stage for a potential seismic shift in how AI systems are powered.
The new chip, currently in test phases within recommendation engines on platforms like Facebook and Instagram, signals a determined pivot. In the words of Meta’s Chief Product Officer, Chris Cox, the journey is cautious yet promising. The challenge is monumental—one misstep in a field where previous attempts had led to expensive dependencies could set the company back substantially. This story resonates with countless innovators who have taken risks at the frontier of technology, and it reminds me of the adage:
“AI is a reflection of the human mind—both its brilliance and its flaws.” – Sherry Turkle, Professor at MIT
Meta’s approach is a demonstration of how persistent innovation combined with strategic partnerships can disrupt established norms. One can’t help but draw parallels with earlier tech revolutions where custom hardware solutions often precipitated leaps in performance and cost efficiency. For a deeper dive into related industry dynamics, you may find insights in our article on Nvidia's computational challenges as well as our feature on AI innovations in gaming.
GPU Shortages and the Pressure on AI Giants
Even as Meta charges forward with its hardware revolution, both Meta and OpenAI are facing serious capacity constraints with AI chips—specifically GPUs. At a critical juncture where generative AI projects are scaling rapidly, the shortage of these critical components poses a significant bottleneck. Analysts, including those at Morgan Stanley, have highlighted that such capacity constraints are not a distant worry but a present and pressing issue.
The situation is layered. Meta’s continued reliance on GPUs for training expansive models and refining content ranking systems means that any shortage can directly impact user experiences, advertising efficiencies, and overall platform performance. Similarly, OpenAI has reported that its GPU supply is “completely saturated,” a confirmation that even a leading AI research organization is struggling with the same supply chain challenges. It’s an industry-wide reminder that supply chain innovations and diversifications are urgently required to keep pace with the explosive demand for AI.
What this situation also underlines is a broader strategic imperative: companies must consider alternative approaches. OpenAI, for instance, has turned to generating synthetic data with their existing computational infrastructure to bypass some of these limitations. This diversification in strategy is crucial when you consider the pace of growth in AI-dependent applications and the increasing values placed on efficiency and cost reduction.
Cybersecurity Concerns in the AI Landscape
Gridlocked supply chains and hardware innovations are not the only challenges on the radar. In a move that underscores the complex interplay between technology and national security, attorneys general from 21 states, including South Carolina and Georgia, have taken firm action against the controversial Chinese AI application DeepSeek. This software has raised serious concerns about cybersecurity because of its intrusive capabilities—logging chat histories, keystrokes, and search queries, potentially exposing sensitive data to foreign adversaries.
The legislative momentum is building to ensure that government devices, which handle classified and sensitive information, are insulated from these risks. The push for the “No DeepSeek on Government Devices Act” is reminiscent of previous legislative moves such as those targeting social media apps that posed privacy concerns in government environments. Countries like Canada and Australia have already taken steps in this direction, reflecting a growing global consensus on the need for stringent cybersecurity measures in the AI domain.
This collaborative initiative across state lines underscores how cybersecurity and AI are increasingly interwoven in policy discussions. Intellectually, this move parallels efforts in other tech sectors where safeguarding data has become as critical as technological advancement. For those intrigued by the interplay between AI advancements and cybersecurity challenges, explore more about cybersecurity trends in our piece on Misinformation and the Pursuit of AI Truths.
The Escalating Demand for AI Computation Power
Another strand of the sprawling AI narrative is the relentless demand for higher computational power. During a recent discussion led by Cerebras CEO, it was emphasized that the underlying infrastructure powering AI must evolve at an extraordinary pace to keep up with the innovation around it. This perspective is increasingly echoed throughout the industry: as AI systems become more complex, the supporting hardware must also scale accordingly.
The race for computing power encapsulates not only the creation of more efficient chips but also the broader transformation of data centers, cloud infrastructures, and specialized hardware ecosystems. The challenges are especially acute for companies like NVIDIA, whose upcoming GPU Technology Conference (GTC 2025) is expected to highlight groundbreaking solutions and new product iterations, like the anticipated upgrades to their Blackwell GPUs.
For many in the tech industry, these conferences represent a beacon of hope—a promise that continued investment in processing power will enable both incremental and transformative leaps in AI performance. This year, all eyes will be on CEO Jensen Huang’s keynote, which comes at a time when NVIDIA is under added pressure to catalyze solid, impactful changes. His performance could be a turning point given the uncertain market sentiments following some recent product hiccups.
When discussing the future needs for computational capacity, I’m reminded of Vladimir Putin’s assertion, “Artificial intelligence is the future, not only for Russia but for all humankind." The words serve as a testament to the pervasive belief that efficient hardware is the backbone of AI’s next evolutionary leap. For additional insights on this theme, you might like to check out our detailed analysis on Nvidia’s computational demands.
Scaling AI for Real Impact in the Enterprise
At the intersection of innovation and everyday application lies the transformative power of AI in businesses. Enterprises are increasingly integrating AI into their operations, not as an experiment but as a fully scaled, strategic approach to reinventing how business is conducted. From automating mundane tasks to delivering enhanced customer experiences and reimagining data-driven decision making, AI is rapidly transforming the landscape of enterprise operations.
In our evolving digital world, scale matters. Many companies have moved past pilot projects to deploy AI systems that can handle massive datasets, streamline logistics, and free human resources for more strategic tasks. This shift isn’t merely a technological upgrade—it’s a cultural transformation within organizations. The integration of AI has led to the development of custom, in-house tools that provide competitive advantages and operational efficiencies. For example, by automating repetitive processes, teams can focus on what really matters: innovation and strategic initiatives that drive growth.
Furthermore, the transition to AI-powered environments is fostering a culture of continuous improvement. It encourages enterprises to rethink traditional business models, identify new revenue opportunities, and even explore untapped markets. The shift from being reactive to proactive relies on making decisions at the speed of data, a journey that many forward-thinking companies are embracing wholeheartedly. Our article on AI in Action: How Enterprises Are Scaling AI for Real Business Impact provides extensive examples of just how much this transformation is influencing various sectors.
Rethinking Data Center Efficiency: The Quest for Better Storage Solutions
While much of the industry is preoccupied with processing power, there is another critical challenge that executives and engineers must overcome—data storage. As hard disk drives (HDDs) grow larger, a critical metric like input/output efficiency declines, jeopardizing the pace at which data centers can deliver performance. Meta’s engineers, grappling with this issue, have brought attention to the limitations of traditional HDDs in the context of data center workloads.
Meta envisions a future where the introduction of QLC (quad-level cell) flash storage offers a middle ground. QLC flash promises high-density storage that can handle the intensive demands characteristic of modern AI applications, thereby bridging the gap between cost-effective HDDs and performance-centric TLC (triple-level cell) flash. Despite its challenges with capacity and endurance, technological advancements like the 2Tb QLC NAND die are starting to tip the scales.
This hardware evolution has far-reaching implications. For vast platforms that process enormous amounts of data—be it social media feeds, recommendation systems, or enterprise operations—optimizing storage not only boosts performance but also offers significant energy savings. As highlighted in a recent discussion by Meta’s engineers, the progress in QLC storage could elevate data center operations to a whole new level, reconfiguring the traditional models of storage deployment. Those fascinated by the intricacies of data center evolution can explore further insights in our related technology-focused coverage.
Interconnecting the Threads: A Comprehensive AI Ecosystem
The narratives emerging from Meta’s chip ambitions, the global scramble for GPUs, and the proactive cybersecurity measures illustrate that we’re witnessing a broader metamorphosis in the AI ecosystem. What binds these stories together is the recognition that the evolution of artificial intelligence is overwhelmingly multifaceted. It is not solely about creating smarter AI algorithms but also about empowering those algorithms with the right hardware, ensuring data safety, and scaling these breakthroughs to impact everyday businesses.
It’s intriguing to observe how innovations in one area can ripple into another. Meta’s new chip, for example, doesn’t just promise lower costs—it hints at an industry on the cusp of rethinking the hardware-software division entirely. Meanwhile, the constraints in GPU supply are prompting organizations to explore alternative methods, such as synthetic data generation or even low-cost AI models that can perform key functions efficiently. Similarly, cybersecurity challenges necessitate that both policy makers and tech companies remain agile, altering regulations to ensure the safe and ethical deployment of AI technologies.
One could liken this to a beautifully choreographed dance, where each step, if executed in harmony, can lead to a significant breakthrough. The advancements in data storage technology, highlighted by Meta’s drive to harness the potential of QLC, reveal that optimizing one piece of the puzzle can have cascading benefits across technology platforms. This interconnectedness mirrors a broader principle found time and again in technology: to achieve transformative change, every component must evolve together.
For those who are eager to see how these threads come together, our recent updates on topics such as AI innovations in gaming and discussions on emerging hardware trends provide further layers of perspective to the rapidly shifting AI landscape.
Looking Ahead: The Road to AI Maturity
While the challenges are clear—ranging from the need for affordable, efficient chips to overcoming hardware limitations and tightening cybersecurity—the commitment shown by industry leaders suggests a bright future. AI, after all, is more than a technological advancement; it’s a testament to the collective pursuit of innovation and growth. The stories unfolding from Meta, OpenAI, NVIDIA, and various enterprise initiatives indicate a sector that is not only resilient but also driven by an exciting vision of what’s next.
As we dissect these developments and gauge their impact, it’s important to remember that every stride forward demands both bold vision and meticulous execution. The ambition to produce state-of-the-art hardware and software solutions is a continuous journey—a journey that has the potential to redefine the boundaries of what AI can achieve. Whether it’s through custom chips that challenge incumbents, scaling operations in businesses, or ushering in new paradigms in data storage, the road ahead is laden with possibilities.
This evolution is not confined to a single region or company. Innovations are globally interconnected, as evidenced by legislative actions in the United States against potential cybersecurity threats, and advancements in computational technologies showcased at conferences that attract a worldwide audience. The global nature of AI underscores the collective challenge and the shared opportunity—a challenge that invites collaboration, research, and a steadfast commitment to ethical and sustainable growth in technology.
It is these multifaceted endeavors that will define the next chapter in AI history. For anyone interested in the intersection of technology, business, and policy, the coming months are sure to be full of intriguing developments and dynamic shifts in the industry landscape.
Further Readings
- Meta's Secret AI Weapon: The Chip That Could Break Nvidia's Grip – Yahoo Finance
- S.C., Ga. Attorneys General Fight Chinese Cyberspying Through AI App – WRDW
- Meta and OpenAI Say They Don't Have Enough AI Chips – Yahoo Finance
- Watch AI Needs More Power, Cerebras CEO Says – Bloomberg
- How to Watch the NVIDIA GTC 2025 Keynote with CEO Jensen Huang – Engadget
- AI in Action: How Enterprises Are Scaling AI for Real Business Impact – CIO
- Facebook Engineers on the Impact of Bigger Hard Disk Drives – TechRadar
In our ever-evolving digital era, these converging trends underscore an undeniable truth: the future of artificial intelligence is being written today, one breakthrough at a time.